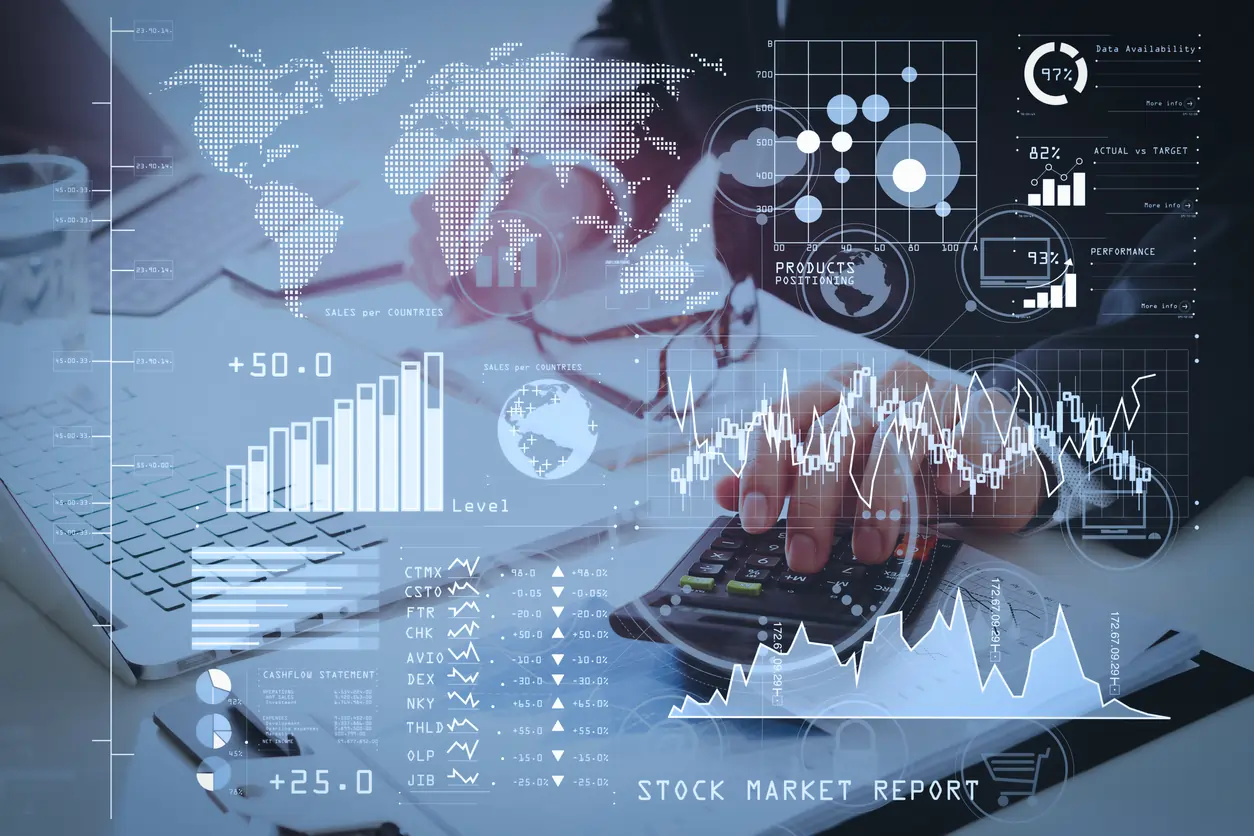
The Role of AI in Predictive Analysis for Finance
In the ever-evolving landscape of finance, the integration of Artificial Intelligence (AI) has become increasingly prominent, particularly in predictive analysis. AI-driven predictive analysis offers invaluable insights for financial institutions, investors, and individuals alike, enabling more informed decision-making and risk management. This blog post delves into the significance of AI in predictive analysis for finance, its applications, challenges, and future prospects.
Understanding Predictive Analysis
Predictive analysis involves the use of data, statistical algorithms, and machine learning techniques to identify the likelihood of future outcomes based on historical data. In finance, predictive analysis plays a crucial role in forecasting market trends, assessing investment risks, detecting fraudulent activities, and optimizing trading strategies.
The Emergence of AI in Finance
Artificial Intelligence has revolutionized the financial sector by enhancing the capabilities of predictive analysis. Machine learning algorithms, powered by AI, can analyze vast amounts of data with remarkable speed and accuracy, uncovering patterns and correlations that human analysts may overlook. This enables financial institutions to make data-driven decisions in real-time, leading to improved profitability and risk mitigation.
Applications of AI in Predictive Analysis for Finance
- Stock Market Forecasting:
AI-powered predictive models analyze historical stock prices, trading volumes, market sentiment, and other relevant factors to forecast future price movements. These models help investors identify potential investment opportunities and optimize portfolio management strategies.
- Credit Risk Assessment:
Financial institutions utilize AI-driven predictive analysis to assess the creditworthiness of loan applicants. By analyzing various data points such as credit history, income levels, and spending patterns, AI models can accurately predict the likelihood of default, enabling lenders to make informed lending decisions.
- Fraud Detection:
AI algorithms can detect suspicious patterns and anomalies in financial transactions, flagging potential instances of fraud or money laundering. By continuously learning from new data, these models improve their accuracy over time, reducing the financial losses associated with fraudulent activities.
- Algorithmic Trading:
AI-driven predictive analysis powers algorithmic trading systems that execute trades based on predefined criteria and market conditions. These systems can analyze large datasets and execute trades at high speeds, capitalizing on market inefficiencies and generating profits for investors.
Challenges and Limitations
Despite its numerous benefits, AI-driven predictive analysis in finance is not without challenges and limitations. Some of the key challenges include:
- Data Quality and Availability:
The effectiveness of AI models heavily depends on the quality and availability of data. Inaccurate or incomplete data can lead to biased predictions and unreliable outcomes.
- Model Interpretability:
AI models often operate as black boxes, making it challenging for analysts to interpret their decisions. Lack of transparency can undermine trust in the predictive analysis process, especially in highly regulated industries like finance.
- Overfitting and Generalization:
AI models may suffer from overfitting, where they perform well on historical data but fail to generalize to new, unseen data. Achieving a balance between model complexity and generalization remains a significant challenge in predictive analysis.
Future Prospects
Despite these challenges, the future of AI in predictive analysis for finance looks promising. Advancements in AI technology, coupled with increasing access to big data, are expected to drive further innovation in this field. As AI algorithms become more sophisticated and capable of handling complex financial data, their applications in predictive analysis will continue to expand, empowering financial institutions and investors with actionable insights and decision-making capabilities.
Frequently Asked Questions (FAQs)
Q1: How accurate are AI-driven predictive models in finance?
A1: The accuracy of AI-driven predictive models varies depending on various factors such as data quality, model complexity, and the specific application. However, studies have shown that AI models can outperform traditional statistical methods in predicting financial outcomes.
Q2: Can AI predict stock market crashes?
A2: While AI models can analyze historical data and identify patterns associated with market downturns, predicting stock market crashes with absolute certainty remains challenging. Market dynamics are influenced by numerous unpredictable factors, making accurate predictions of crashes difficult.
Q3: Is AI replacing human analysts in finance?
A3: AI is augmenting rather than replacing human analysts in finance. While AI-driven predictive analysis can automate repetitive tasks and enhance efficiency, human expertise is still essential for interpreting results, making strategic decisions, and ensuring regulatory compliance.
Q4: How can businesses leverage AI for predictive analysis in finance?
A4: Businesses can leverage AI by investing in data infrastructure, developing customized AI models tailored to their specific needs, and integrating AI-powered analytics tools into their decision-making processes. Collaboration between data scientists, financial analysts, and domain experts is crucial for successful implementation.
Conclusion
In conclusion, AI-driven predictive analysis is reshaping the landscape of finance, offering unprecedented opportunities for informed decision-making and risk management. By harnessing the power of AI algorithms, financial institutions and investors can gain actionable insights, optimize strategies, and stay ahead in an increasingly competitive market environment. While challenges exist, the continued advancements in AI technology hold immense promise for the future of predictive analysis in finance.
Expanding on the Applications of AI in Predictive Analysis
- Customer Relationship Management (CRM):
AI plays a vital role in enhancing customer relationship management in the financial sector. Predictive analytics powered by AI can analyze customer data, including transaction history, communication patterns, and feedback, to predict customer behavior and preferences. This allows financial institutions to tailor their marketing strategies, improve customer service, and foster long-term relationships with clients.
- Portfolio Management:
AI-driven predictive analysis is instrumental in portfolio management, helping investors optimize their investment strategies and maximize returns. By analyzing historical market data, economic indicators, and asset performance, AI algorithms can recommend portfolio allocations, asset diversification strategies, and risk management techniques tailored to individual investor preferences and financial goals.
- Regulatory Compliance:
In an increasingly regulated financial environment, AI-driven predictive analysis aids financial institutions in ensuring compliance with regulatory requirements. AI models can analyze vast amounts of regulatory data, identify compliance risks, and flag potential violations, enabling proactive measures to mitigate legal and regulatory risks. This helps financial institutions avoid costly fines, penalties, and reputational damage associated with non-compliance.
Exploring the Ethical Implications of AI in Predictive Analysis
While AI-driven predictive analysis offers numerous benefits for the finance industry, it also raises important ethical considerations. The use of AI algorithms in decision-making processes, particularly in areas such as credit scoring and loan approvals, can perpetuate biases and discrimination inherent in the underlying data. For example, if historical data reflects discriminatory practices, AI models may inadvertently replicate and amplify these biases, leading to unfair outcomes for certain demographic groups. Therefore, it is essential for financial institutions to implement safeguards and ethical guidelines to mitigate bias and ensure fairness and transparency in AI-driven predictive analysis.
Addressing the Skills Gap in AI and Predictive Analysis
As the demand for AI-driven predictive analysis continues to grow in the finance industry, there is a pressing need for skilled professionals with expertise in data science, machine learning, and finance. Financial institutions must invest in training programs and educational initiatives to bridge the skills gap and equip their workforce with the necessary knowledge and skills to harness the power of AI effectively. By fostering a culture of continuous learning and innovation, financial institutions can stay at the forefront of AI-driven predictive analysis and drive sustainable growth and competitive advantage in the dynamic landscape of finance.
Conclusion
In conclusion, AI-driven predictive analysis holds immense potential to revolutionize the finance industry, offering unprecedented opportunities for informed decision-making, risk management, and strategic innovation. By leveraging the power of AI algorithms, financial institutions can gain actionable insights, optimize operations, and drive business growth in an increasingly complex and competitive market environment. However, to fully realize the benefits of AI-driven predictive analysis, it is essential for financial institutions to address the challenges, ethical considerations, and skills gap associated with its implementation. With the right strategies and investments, AI-driven predictive analysis can empower financial institutions to navigate uncertainty, capitalize on opportunities, and achieve sustainable success in the digital age.